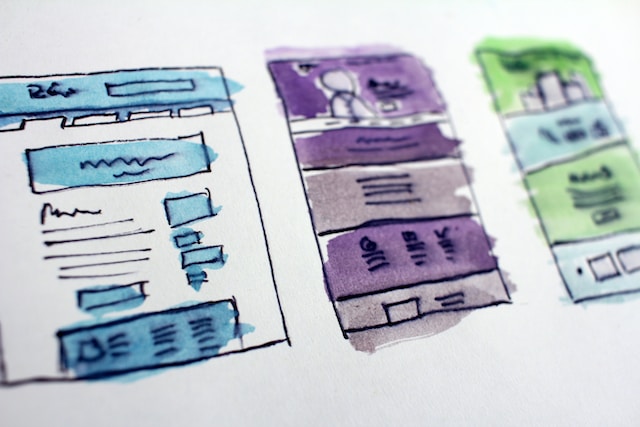
This is a guest post by Rachael G. Samberg, Timothy Vollmer, and Samantha Teremi, professionals within the Office of Scholarly Communication Services at UC Berkeley Library.
On academic and library listservs, there has emerged an increasingly fraught discussion about licensing scholarly content when scholars’ research methodologies rely on artificial intelligence (AI). Scholars and librarians are rightfully concerned that non-profit educational research methodologies like text and data mining (TDM) that can (but do not necessarily) incorporate usage of AI tools are being clamped down upon by publishers. Indeed, libraries are now being presented with content license agreements that prohibit AI tools and training entirely, irrespective of scholarly purpose.
Conversely, publishers, vendors, and content creators—a group we’ll call “rightsholders” here—have expressed valid concerns about how their copyright-protected content is used in AI training, particularly in a commercial context unrelated to scholarly research. Rightsholders fear that their livelihoods are being threatened when generative AI tools are trained and then used to create new outputs that they believe could infringe upon or undermine the market for their works.
Within the context of non-profit academic research, rightsholders’ fears about allowing AI training, and especially non-generative AI training, are misplaced. Newly-emerging content license agreements that prohibit usage of AI entirely, or charge exorbitant fees for it as a separately-licensed right, will be devastating for scientific research and the advancement of knowledge. Our aim with this post is to empower scholars and academic librarians with legal information about why those licensing outcomes are unnecessary, and equip them with alternative licensing language to adequately address rightsholders’ concerns.
To that end, we will:
- Explain the copyright landscape underpinning the use of AI in research contexts;
- Address ways that AI usage can be regulated to protect rightsholders, while outlining opportunities to reform contract law to support scholars; and
- Conclude with practical language that can be incorporated into licensing agreements, so that libraries and scholars can continue to achieve licensing outcomes that satisfy research needs.
Our guidance is based on legal analysis as well as our views as law and policy experts working within scholarly communication. While your mileage or opinions may vary, we hope that the explanations and tools we provide offer a springboard for discussion within your academic institutions or communities about ways to approach licensing scholarly content in the age of AI research.
Copyright and AI training
As we have recently explored in presentations and posts, the copyright law and policy landscape underpinning the use of AI models is complex, and regulatory decision-making in the copyright sphere will have ramifications for global enterprise, innovation, and trade. A much-discussed group of lawsuits and a parallel inquiry from the U.S. Copyright Office raise important and timely legal questions, many of which we are only beginning to understand. But there are two precepts that we believe are clear now, and that bear upon the non-profit education, research, and scholarship undertaken by scholars who rely on AI models.
First, as the UC Berkeley Library has explained in greater detail to the Copyright Office, training artificial intelligence is a fair use—and particularly so in a non-profit research and educational context. (For other similar comments provided to the Copyright Office, see, e.g., the submissions of Authors Alliance and Project LEND). Maintaining its continued treatment as fair use is essential to protecting research, including TDM.
TDM refers generally to a set of research methodologies reliant on computational tools, algorithms, and automated techniques to extract revelatory information from large sets of unstructured or thinly-structured digital content. Not all TDM methodologies necessitate usage of AI models in doing so. For instance, the words that 20th century fiction authors use to describe happiness can be searched for in a corpus of works merely by using algorithms looking for synonyms and variations of words like “happiness” or “mirth,” with no AI involved. But to find examples of happy characters in those books, a researcher would likely need to apply what are called discriminative modeling methodologies that first train AI on examples of what qualities a happy character demonstrates or exhibits, so that the AI can then go and search for occurrences within a larger corpus of works. This latter TDM process involves AI, but not generative AI; and scholars have relied non-controversially on this kind of non-generative AI training within TDM for years.
Previous court cases like Authors Guild v. HathiTrust, Authors Guild v. Google, and A.V. ex rel. Vanderhye v. iParadigms have addressed fair use in the context of TDM and confirmed that the reproduction of copyrighted works to create and conduct text and data mining on a collection of copyright-protected works is a fair use. These cases further hold that making derived data, results, abstractions, metadata, or analysis from the copyright-protected corpus available to the public is also fair use, as long as the research methodologies or data distribution processes do not re-express the underlying works to the public in a way that could supplant the market for the originals.
For the same reasons that the TDM processes constitute fair use of copyrighted works in these contexts, the training of AI tools to do that text and data mining is also fair use. This is in large part because of the same transformativeness of the purpose (under Fair Use Factor 1) and because, just like “regular” TDM that doesn’t involve AI, AI training does not reproduce or communicate the underlying copyrighted works to the public (which is essential to the determination of market supplantation for Fair Use Factor 4).
But, while AI training is no different from other TDM methodologies in terms of fair use, there is an important distinction to make between the inputs for AI training and generative AI’s outputs. The overall fair use of generative AI outputs cannot always be predicted in advance: The mechanics of generative AI models’ operations suggest that there are limited instances in which generative AI outputs could indeed be substantially similar to (and potentially infringing of) the underlying works used for training; this substantial similarity is possible typically only when a training corpus is rife with numerous copies of the same work. And a recent case filed by the New York Times addresses this potential similarity problem with generative AI outputs.
Yet, training inputs should not be conflated with outputs: The training of AI models by using copyright-protected inputs falls squarely within what courts have already determined in TDM cases to be a transformative fair use. This is especially true when that AI training is conducted for non-profit educational or research purposes, as this bolsters its status under Fair Use Factor 1, which considers both transformativeness and whether the act is undertaken for non-profit educational purposes.
Were a court to suddenly determine that training AI was not fair use, and AI training was subsequently permitted only on “safe” materials (like public domain works or works for which training permission has been granted via license), this would curtail freedom of inquiry, exacerbate bias in the nature of research questions able to be studied and the methodologies available to study them, and amplify the views of an unrepresentative set of creators given the limited types of materials available with which to conduct the studies.
The second precept we uphold is that scholars’ ability to access the underlying content to conduct fair use AI training should be preserved with no opt-outs from the perspective of copyright regulation.
The fair use provision of the Copyright Act does not afford copyright owners a right to opt out of allowing other people to use their works in any other circumstance, for good reason: If content creators were able to opt out of fair use, little content would be available freely to build upon. Uniquely allowing fair use opt-outs only in the context of AI training would be a particular threat for research and education, because fair use in these contexts is already becoming an out-of-reach luxury even for the wealthiest institutions. What do we mean?
In the U.S., the prospect of “contractual override” means that, although fair use is statutorily provided for, private parties like publishers may “contract around” fair use by requiring libraries to negotiate for otherwise lawful activities (such as conducting TDM or training AI for research). Academic libraries are forced to pay significant sums each year to try to preserve fair use rights for campus scholars through the database and electronic content license agreements that they sign. This override landscape is particularly detrimental for TDM research methodologies, because TDM research often requires use of massive datasets with works from many publishers, including copyright owners who cannot be identified or who are unwilling to grant such licenses.
So, if the Copyright Office or Congress were to enable rightsholders to opt-out of having their works fairly used for training AI for scholarship, then academic institutions and scholars would face even greater hurdles in licensing content for research. Rightsholders might opt out of allowing their work to be used for AI training fair uses, and then turn around and charge AI usage fees to scholars (or libraries)—essentially licensing back fair uses for research.
Fundamentally, this undermines lawmakers’ public interest goals: It creates a risk of rent-seeking or anti-competitive behavior through which a rightsholder can demand additional remuneration or withhold granting licenses for activities generally seen as being good for public knowledge or that rely on exceptions like fair use. And from a practical perspective, allowing opt-outs from fair uses would impede scholarship by or for research teams who lack grant or institutional funds to cover these additional licensing expenses; penalize research in or about underfunded disciplines or geographical regions; and result in bias as to the topics and regions that can be studied.
“Fair use” does not mean “unregulated”
Although training AI for non-profit scholarly uses is fair use from a copyright perspective, we are not suggesting AI training should be unregulated. To the contrary, we support guardrails because training AI can carry risk. For example, researchers have been able to use generative AI like ChatGPT to solicit personal information by bypassing platform safeguards.
To address issues of privacy, ethics, and the rights of publicity (which govern uses of people’s voices, images, and personas), there should be the adoption of best practices, private ordering, and other regulations.
For instance, as to best practices, scholar Matthew Sag has suggested preliminary guidelines to avoid violations of privacy and the right to publicity. First, he recommends that AI platforms avoid training their large language models on duplicates of the same work. This would reduce the likelihood that the models could produce copyright-infringing outputs (due to memorization concerns), and it would also lessen the likelihood that any content containing potentially private or sensitive information would be outputted from having been fed into the training process multiple times. Second, Sag suggests that AI platforms engage in “reinforcement learning through human feedback” when training large language models. This practice could cut down on privacy or rights of publicity concerns by involving human feedback at the point of training, instead of leveraging filtering at the output stage.
Private ordering would rely on platforms or communities to implement appropriate policies governing privacy issues, rights of publicity, and ethical concerns. For example, the UC Berkeley Library has created policies and practices (called “Responsible Access Workflows”) to help it make decisions around whether—and how—special collection materials may be digitized and made available online. Our Responsible Access Workflows require review of collection materials across copyright, contracts, privacy, and ethics parameters. Through careful policy development, the Library applies an ethics of care approach to making available online the collection content with ethical concerns. Even if content is not shared openly online, it doesn’t mean it’s unavailable for researchers for use in person; we simply have decided not to make that content available in digital formats with lower friction for use. We aim to apply transparent information about our decision-making, and researchers must make informed decisions about how to use the collections, whether or not they are using them in service of AI.
And finally, concerning regulations, countries like those in the EU have recently introduced an AI training framework that requires, among other things, the disclosure of source content, and the rights for content creators to opt out of having their works included in training sets except when the AI training is being done for research purposes by research organizations, cultural heritage institutions, and their members or scholars. United States agencies could consider implementing similar regulations here.
But from a copyright perspective, and within non-profit academic research, fair use in AI training should be preserved without the opportunity to opt out for the reasons we discuss above. Such an approach regarding copyright would also be consistent with the distinction the EU has made for AI training in academic settings, as the EU’s Digital Single Market Directive bifurcates practices outside the context of scholarly research.
While we favor regulation that preserves fair use, it is also important to note that merely preserving fair use rights in scholarly contexts for training AI is not the end of the story in protecting scholarly inquiry. So long as the United States permits contractual override of fair uses, libraries and researchers will continue to be at the mercy of publishers aggregating and controlling what may be done with the scholarly record, even if authors dedicate their content to the public domain or apply a Creative Commons license to it. So in our view, the real work that should be done is pursuing legislative or regulatory arrangements like the approximately 40 other countries that have curtailed the ability of contracts to abrogate fair use and other limitations and exceptions to copyright within non-profit scholarly and educational uses. This is a challenging, but important, mission.
Licensing guidance in the meantime
While the statutory, regulatory, and private governance landscapes are being addressed, libraries and scholars need ways to preserve usage rights for content when training AI as part of their TDM research methodologies. We have developed sample license language intended to address rightsholders’ key concerns while maintaining scholars’ ability to train AI in text and data mining research. We drafted this language to be incorporated into amendments to existing licenses that fail to address TDM, or into stand-alone TDM and AI licenses; however, it is easily adaptable into agreements-in-chief (and we encourage you to do so).
We are certain our terms can continue to be improved upon over time or be tailored for specific research needs as methodologies and AI uses change. But in the meantime, we think they are an important step in the right direction.
With that in mind, it is important to understand that within contracts applying U.S. law, more specific language controls over general language in a contract. So, even if there is a clause in a license agreement that preserves fair use, if it is later followed by a TDM clause that restricts how TDM can be conducted (and whether AI can be used), then that more specific language governs TDM and AI usage under the agreement. This means that libraries and scholars must be mindful when negotiating TDM and AI clauses as they may be contracting themselves out of rights they would otherwise have had under fair use.
So, how can a library or scholar negotiate sufficient AI usage rights while acknowledging the concerns of publishers? We believe publishers have attempted to curb AI usage because they are concerned about: (1) the security of their licensed products, and the fear that researchers will leak or release content behind their paywall; and (2) AI being used to create a competing product that could substitute for the original licensed product and undermine their share of the market. While these concerns are valid, they reflect longstanding fears over users’ potential generalized misuse of licensed materials in which they do not hold copyright. But publishers are already able to—and do—impose contractual provisions disallowing the creation of derivative products and systematically sharing licensed content with third-parties, so additionally banning the use of AI in doing so is, in our opinion, unwarranted.
We developed our sample licensing language to precisely address these concerns by specifying in the grant of license that research results may be used and shared with others in the course of a user’s academic or non-profit research “except to the extent that doing so would substantially reproduce or redistribute the original Licensed Materials, or create a product for use by third parties that would substitute for the Licensed Materials.” Our language also imposes reasonable security protections in the research and storage process to quell fears of content leakage.
Perhaps most importantly, our sample licensing language preserves the right to conduct TDM using “machine learning” and “other automated techniques” by expressly including these phrases in the definition for TDM, thereby reserving AI training rights (including as such AI training methodologies evolve), provided that no competing product or release of the underlying materials is made.
The licensing road ahead
As legislation and standards around AI continue to develop, we hope to see express contractual allowance for AI training become the norm in academic licensing. Though our licensing language will likely need to adapt to and evolve with policy changes and research or technological advancements over time, we hope the sample language can now assist other institutions in their negotiations, and help set a licensing precedent so that publishers understand the importance of allowing AI training in non-profit research contexts. While a different legislative and regulatory approach may be appropriate in the commercial context, we believe that academic research licenses should preserve the right to incorporate AI, especially without additional costs being passed to subscribing institutions or individual users, as a fundamental element of ensuring a diverse and innovative scholarly record.