This post is part of Fair Use Week series, cross-posted at https://sites.harvard.edu/fair-use-week/2024/02/29/fair-use-week-2024-day-four-with-guest-expert-dave-hansen/
AI programs and their outputs raise all sorts of interesting questions–now found in the form of some 20+ lawsuits, many of them massive class actions.
One of the most important questions is whether it is permissible to use copyrighted works as training data to develop AI models themselves, on top of which AI services like ChatGPT are built (read here for a good overview of the component parts and “supply chain” of generative AI, reviewed through a legal lens).
For the question of fair use of AI training data, you’ll find that almost everyone writing about this question in the US context says the answer turns on two or three precedents–especially the Google Books case and the HathiTrust case–and a concept referred to as “non-expressive use” (or sometimes “non-consumptive use”). This concept of non-expressive use and those cases have proven to be foundational for all sorts of applications that extend well beyond generative AI, including basic web search, plagiarism detection tools, and text and data mining research. Since this idea has received so much attention, I thought this fair use week was a good opportunity to explore what this concept is.
What is non-expressive use?
Non-expressive use refers to uses that involve copying, but don’t communicate the expressive aspects of the work to be read or otherwise enjoyed. It is a term coined, as far as I can tell, by law professor Matthew Sag in a series of papers titled “Copyright and Copyright Reliant Technology” (in which he observes that courts have been approving of such uses–for example in search engine cases–albeit without a coherent framework) and then more directly in “Orphan Works as Grist for the Data Mill” and later in an article titled “The New Legal Landscape for Text Mining and Machine Learning.” You can do much better than this blog post if you just read Matt’s articles. But, since you’re here, the argument is basically built on two propositions:
Proposition #1: “Facts are not copyrightable” is a phrase you’ll hear somewhere near the beginning of the lecture on copyright 101. It, along with the “idea-expression” dichotomy and some related doctrines are some of the ways that copyright law draws a line between protected content and those underlying facts and ideas that anyone is free to use. These protections for free use of facts and ideas are more than just a line in the sand drawn by Congress or the courts. As the U.S. Supreme Court in Eldred v. Ashcroft most recently explained:
“[The]idea/expression dichotomy strike[s] a definitional balance between the First Amendment and the Copyright Act by permitting free communication of facts while still protecting an author’s expression. Due to this distinction, every idea, theory, and fact in a copyrighted work becomes instantly available for public exploitation at the moment of publication.” (citations and quotations omitted).
The law has therefore recognized the distinction between expressive non-expressive works (for example, copyright exists in a novel, but not in a phone book), and that this distinction is so important that the Constitution mandates it. The exact contours of this line have been the subject of a long and not always consistent history, but has slowly come into focus in cases from Baker v. Selden (1879) (“there is a clear distinction between the book, as such, and the art which it is intended to illustrate”) to Feist Publications v. Rural Telephone (1994) (no copyright in telephone white pages).
Proposition #2: Fair use is also one of the Copyright Act’s First Amendment safeguards, per the Supreme Court Eldred. The “transformative use” analysis, in particular, does a lot of work in giving breathing room for others to use existing works in ways that allow for their own criticism and comment. It also has provided ample space for uses that rely on copying to unearth facts and ideas contained within and about underlying works, particularly when doing so in a way that provides a net social benefit.
Transformative use, though not always easy to define in practice, favors uses that avoid substituting for the original expression, but that reuse that content in new ways, with new meaning, message and purpose. While this can apply to downstream expressive uses (e.g., parody is the paradigmatic example that relies on reusing expression itself), its application to non-expressive uses can look even stronger. This is why you find courts like the 9th Circuit in a case about image search saying things like “a search engine may be more transformative than a parody because a search engine provides an entirely new use for the original work, while a parody typically has the same entertainment purpose as the original work,” where search engines copy underlying works primarily for the purpose of helping users discover them.
Fair use for non-expressive use
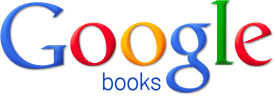
We now have several cases that address non-expressive uses for computational analysis of texts. The three cases, in particular, are iParadigms v. ex rel Vanderhye, in which the Fourth Circuit in 2009 analyzed a plagiarism detection tool that ingested papers and then created a “digital fingerprint” to match them to duplicate content using a statistical technique originally designed to analyze brain waves. The court there concluded that “iParadigms’ use of these works was completely unrelated to expressive content” and therefore constituted transformative fair use. Then in Authors Guild v. HathiTrust and Authors Guild v. Google, we saw the Second Circuit in successive opinions in 2014 and 2015 approve of copying at a massive scale of books used for the purpose of full-text search of those books and related computational, analytical uses. The court, in Google Books, fully briefed on the implications of these projects for computational analysis of texts, explained:
As with HathiTrust (and iParadigms), the purpose of Google’s copying of the original copyrighted books is to make available significant information about those books, permitting a searcher to identify those that contain a word or term of interest, as well as those that do not include reference to it. In addition, through the ngrams tool, Google allows readers to learn the frequency of usage of selected words in the aggregate corpus of published books in different historical periods. We have no doubt that the purpose of this copying is the sort of transformative purpose described in Campbell.
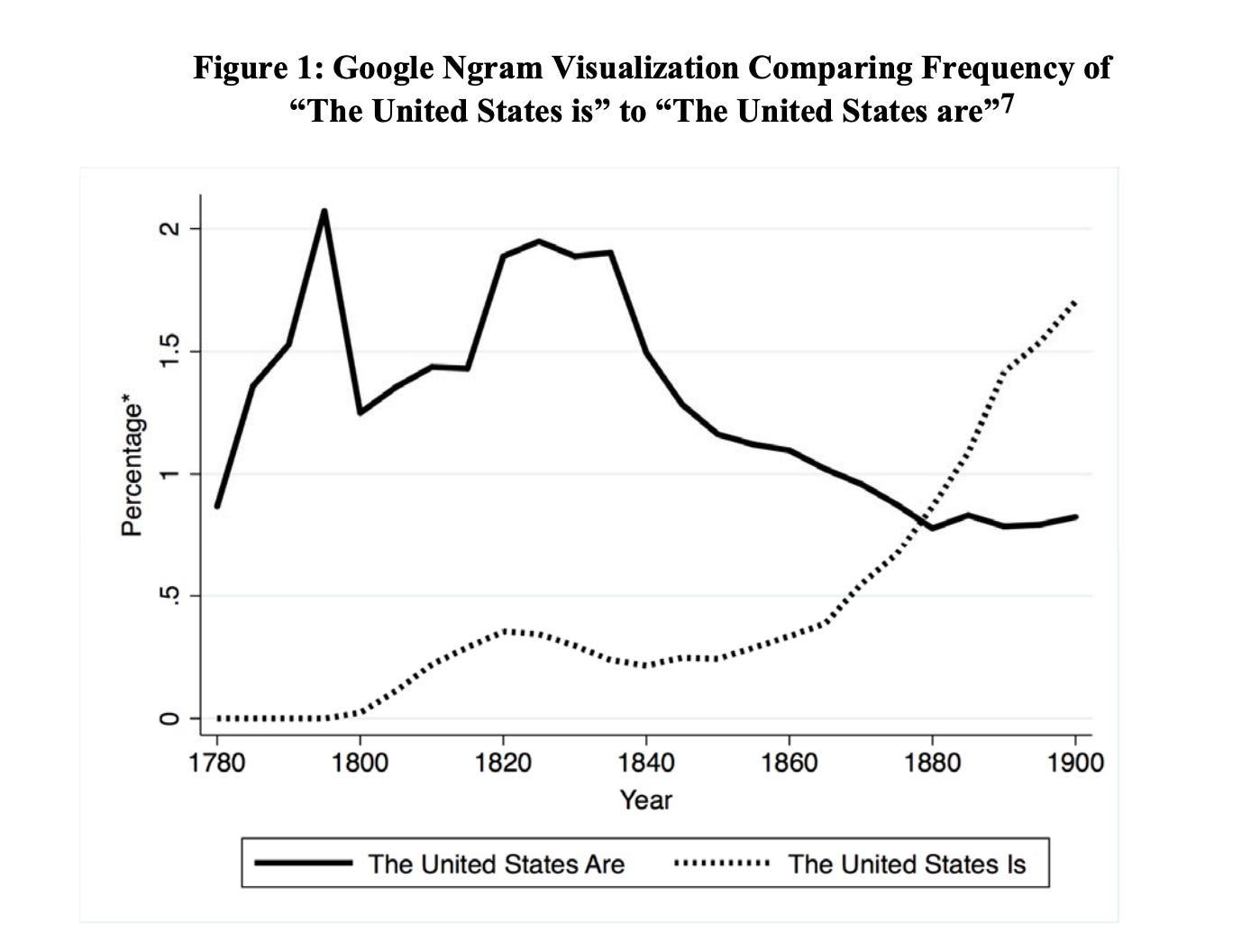
Example from the Digital Humanities Scholars brief in the Google Books case,
illustrating one text mining use enabled by the Google Books corpus.
So, back to AI
There are certainly limits to how much of an underlying work can be described before one crosses the line from non-expressive to substantial use of expressive content. For example, uses that reproduce extensive facts from underlying works to merely repackage content for the same purpose as the original works may face challenges, as in the case of Castlerock Entertainment v. Carol Publishing(about Carol Publishing’s “Seinfeld Aptitude Test” based on facts from the Seinfeld series), which the court concluded as made merely to “repackage Seinfeld to entertain Seinfeld viewers.” And there are real questions (discussed in two excellent recent essays, here and here) about how the law may respond in practice to AI products, particularly ones where outputs look–or at least can be made to look–suspiciously similar to inputs used as training data.
How AI models work is explained much more thoroughly (and much better) elsewhere, but the basic idea is that they are built by developing extraordinarily robust word vectors used to represent the relationships between words. To do this well, these models need to train on a large and diverse set of texts to build out a good model of how humans communicate in a variety of contexts. In short, these copy texts for the purpose of developing a model to describe facts about the underlying works and the relationship of words within them and with each other. What’s new is that we can now do this at a level of complexity and scale almost unimaginable before. Scale and complexity don’t change the underlying principles at issue, however, and so this kind of training seems to me clearly within the bounds of non-expressive use as approved already by the courts in the cases cited above that authors, researchers, and the tech industry have been relying on for nearly a decade.
Discover more from Authors Alliance
Subscribe to get the latest posts sent to your email.